About the Joint Seminar
The UBC/SFU Joint Statistical Seminar is jointly hosted by the graduate students of the UBC Department of Statistics and the SFU Department of Statistics and Actuarial Science. The Spring 2021 event is the second of two events taking place in the 2020/2021 academic year. The event offers Statistics and Actuarial Science graduate and undergraduate students an opportunity to attend seminars with accessible talks about active research areas in the field and provides an opportunity to network with their peers. The Fall 2020 event was organized by graduate students from SFU, and the Spring 2021 event is organized by graduate students from UBC.
The Spring 2021 event includes talks given by six students (three from UBC and three from SFU) and one faculty member from UBC. The event will be held virtually through Zoom this year. Please register for the event through the registration form.
Seminar Schedule
Welcome Message
9:00am - 9:05am
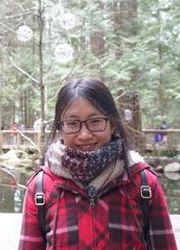
Qiong Zhang (UBC)
Distributed learning of finite Gaussian mixtures
9:05am - 9:30am
Advances in information technology have led to extremely large datasets that are often kept in different storage centers. Existing statistical methods must be adapted to overcome the resulting computational obstacles while retaining statistical validity and efficiency. Split-and-conquer approaches have been applied in many areas, including quantile processes, regression analysis, principal eigenspaces, and exponential families. We study split-and-conquer approaches for the distributed learning of finite Gaussian mixtures. We recommend a reduction strategy and develop an effective MM algorithm. The new estimator is shown to be consistent and retains root-n consistency under some general conditions. Experiments based on simulated and real-world data show that the proposed split-and-conquer approach has comparable statistical performance with the global estimator based on the full dataset if the latter is feasible. It can even slightly outperform the global estimator if the model assumption does not match the real-world data. It also has better statistical and computational performance than some existing methods.
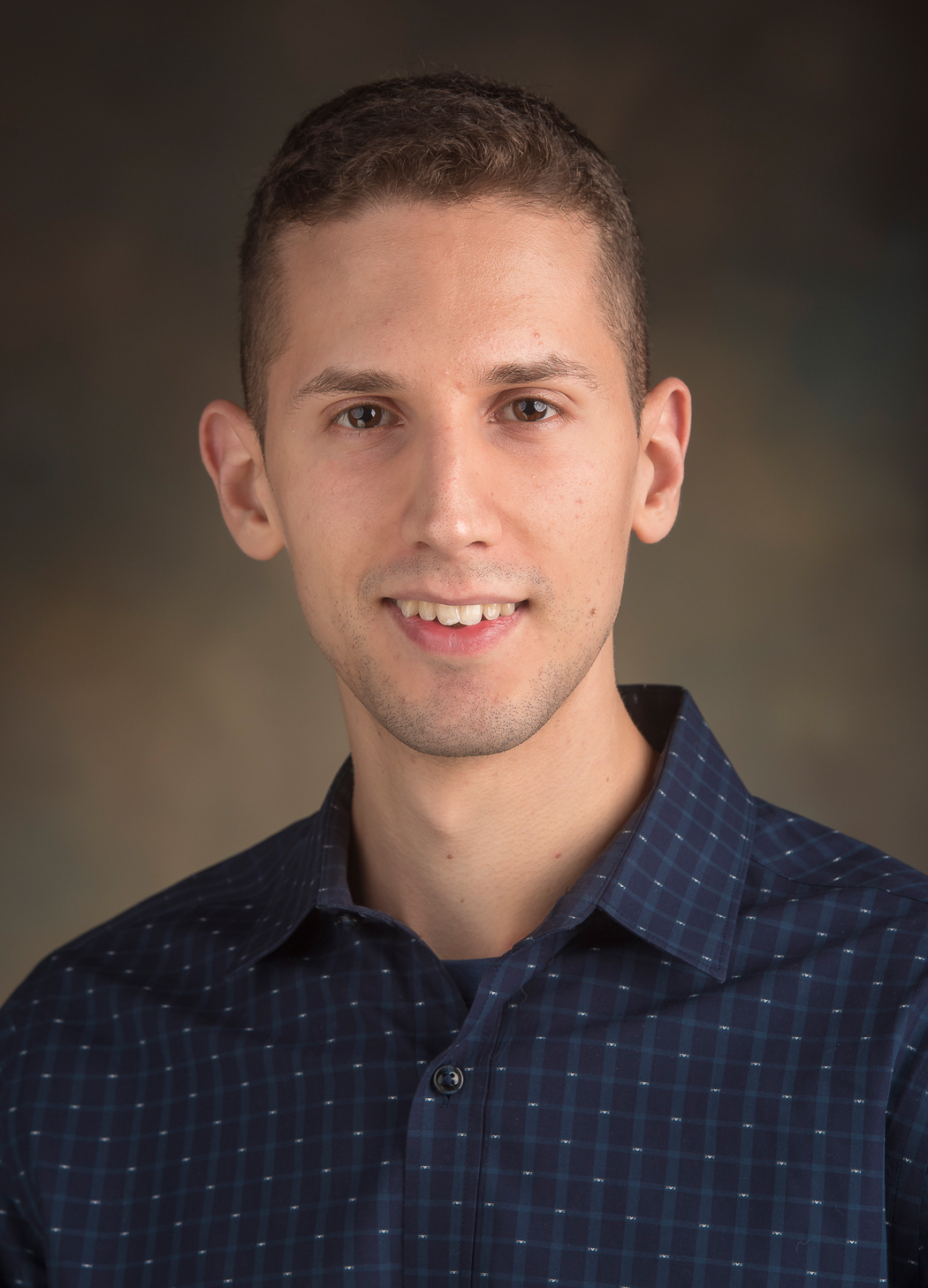
Nikola Surjanovic (SFU)
Using information criteria to improve the performance of tree-based learning algorithms without the use of cross-validation
9:30am - 9:55am
We show that it is possible to prune a regression tree efficiently using information criteria, and we highlight some applications to tree-based ensemble learning methods. Using a modified Bayesian information criterion to prune regression trees without cross-validation, we obtain simplified trees that have prediction accuracy comparable to trees obtained using standard cost-complexity pruning. An extension to random forests that prevents the growth of trees with excessive variance, building upon the work of other authors, is discussed. The extension includes regular random forests as a special case, and is therefore expected to perform at least as well, with a negligible additional computational cost.
Networking Break 1
9:55am - 10:05am
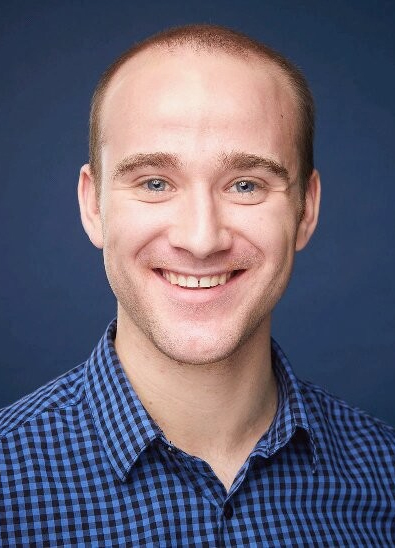
Evan Sidrow (UBC)
Modelling marine mammal sub-dive behaviour with hierarchical hidden Markov models
10:05am - 10:30am
Recent advances in high-frequency tagging technology have made available a vast amount of animal movement data. This rich new data can exhibit simultaneous behavioural processes occurring at different time scales. One can model these processes through a hierarchical hidden Markov model (HHMM), where the system is modelled as a nested structure of hidden Markov models. At very short time scales, however, observations can exhibit complicated dependence structures that cannot be easily captured by traditional HMMs. We demonstrate how to incorporate fine-scale processes into the larger structure of HHMMs while maintaining computational efficiency. We apply our method to dive and accelerometer data collected from northern resident killer whales off the coast of British Columbia, Canada.
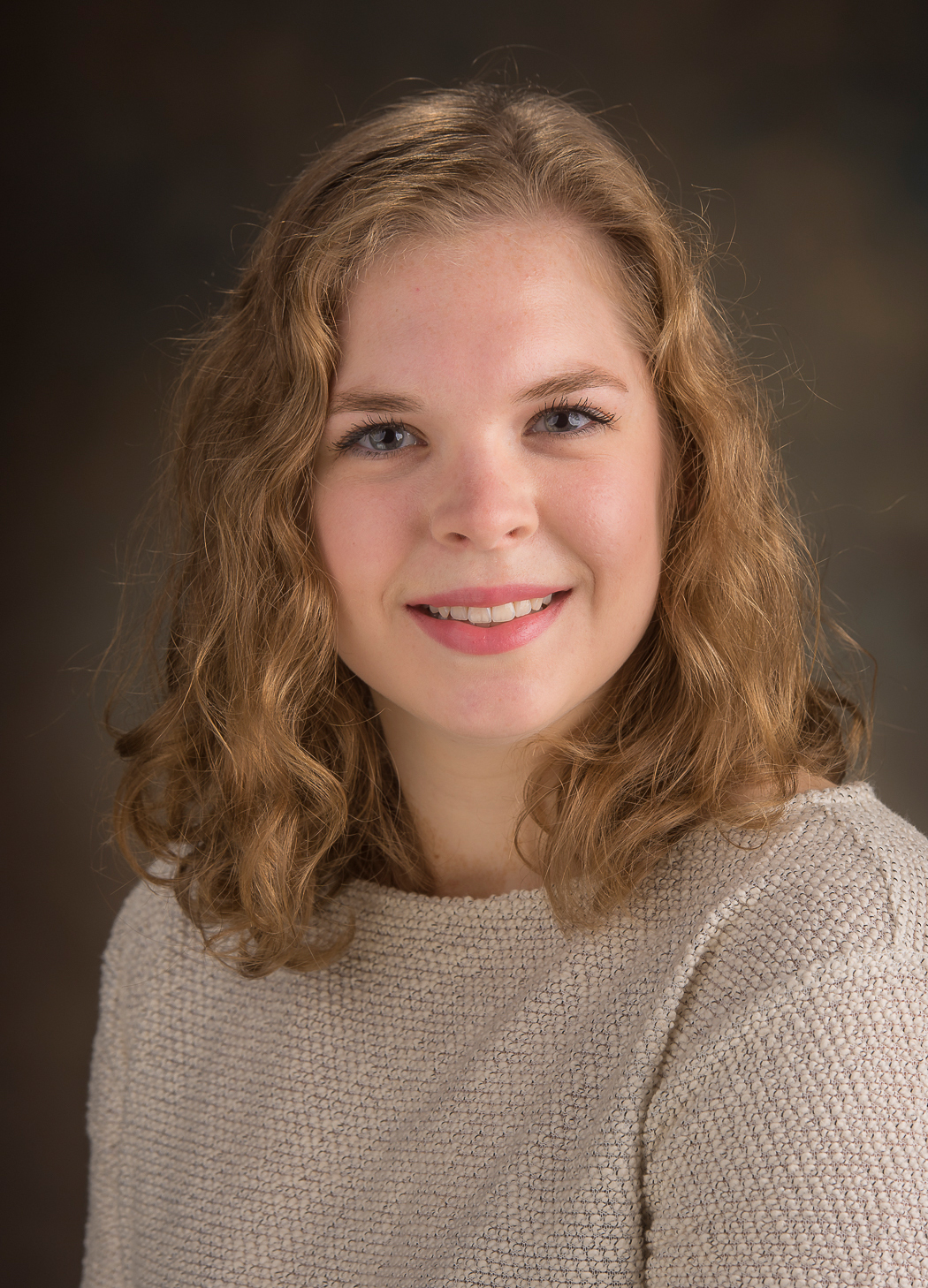
Lisa McQuarrie (SFU)
Autoregressive linear mixed effects models and an application to annual income of breast cancer survivors
10:30am - 10:55am
Yearly observations of annual income are often highly autocorrelated, with the autocorrelation remaining high after adjusting for observed independent variables. Consequently, longitudinal models used to analyze income must allow for residual autocorrelation. We explore two of the most common longitudinal models used to analyze annual income: 1. the autoregressive error model, which is a linear mixed effects model with an AR(1) covariance structure for the error term, and 2. the autoregressive response model, which is a linear mixed effects model that uses lagged values of the response variable as additional explanatory variables. We also contrast these models with a linear mixed effects model with independent errors, to examine if assuming independent errors reduces the goodness of fit. The theoretical properties of these models are explored and illustrated using a simulation study. Additionally, the three models are applied to a data set containing yearly income observations from a sample of breast cancer survivors. We aim to determine the short and long-term effect of a breast cancer diagnosis on a survivor’s annual net income.
Networking Break 2
10:55am - 11:05am
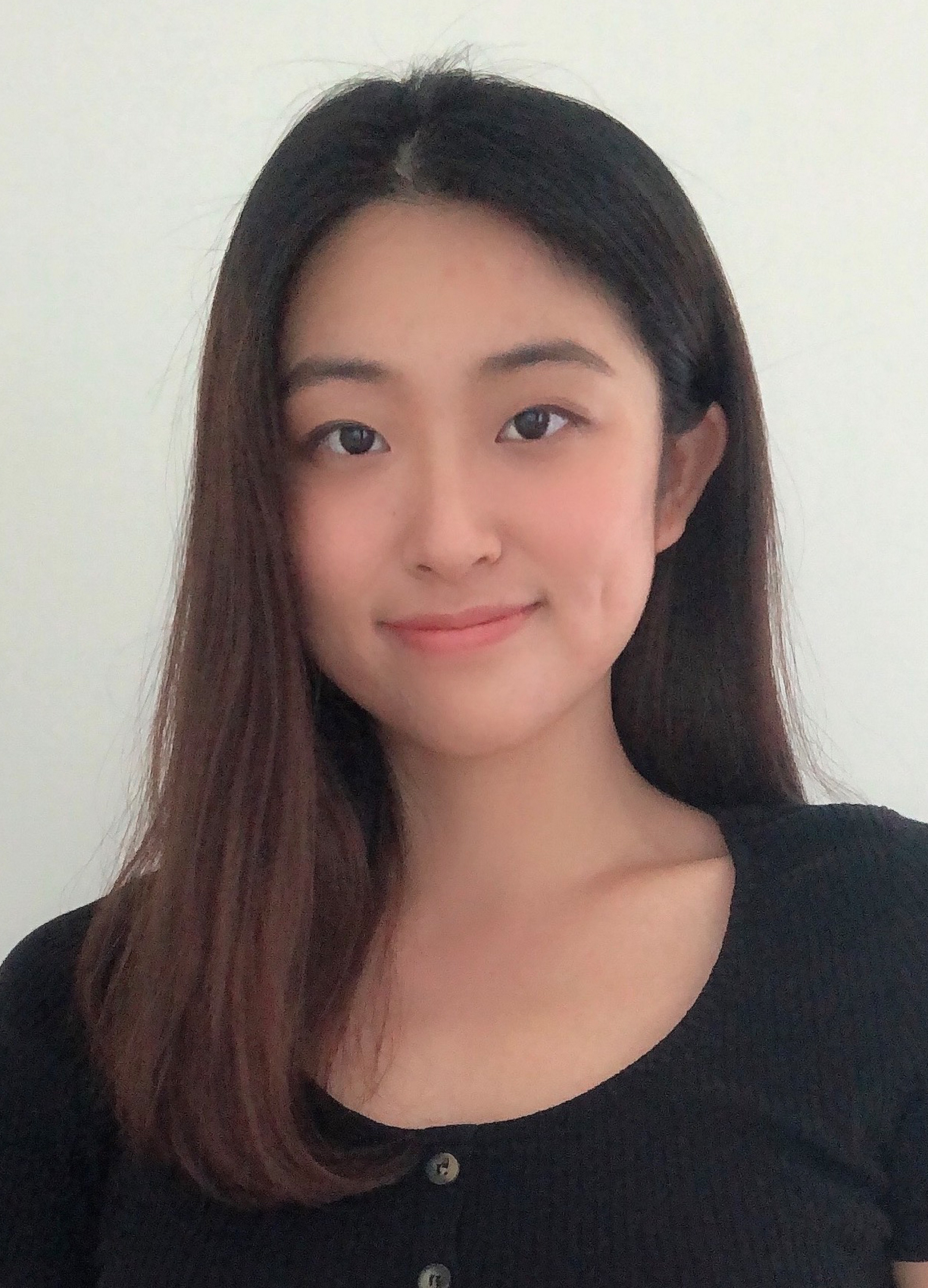
Jiaping(Olivia) Liu (UBC)
A dynamic programming method for a one-dimensional fused Lasso signal approximator
11:05am - 11:30am
Many statistical methods were proposed to solve a fused Lasso signal approximator (FLSA) problem for an exact or approximate solution. However, these methods either provided approximate solutions, not exact solutions of the problem, or yielded the solutions in a relatively long running time. The paper from Nicholas in 2013 proposed a dynamic programming algorithm for a one-dimensional FLSA. It yielded a global optimization (an exact solution) within a linear time complexity in the worst case, which improved the previous methods by solving the exact solution within a lower running time, especially for relatively long sequences. One of the most incredible parts of the paper was its skillful way to handle the knots, which was also the essential point reducing the time complexity. The proposed DP algorithm could also be used for the least squares segmentation, but had a higher time complexity in the worst case.
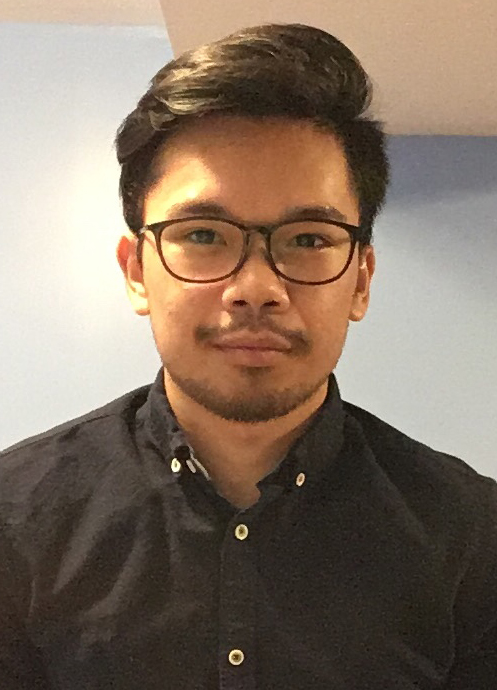
Peter Tea (SFU)
Multilevel models in sports
11:30am - 11:55am
Since the inception of Moneyball, analytics in sport has evolved rapidly — often drawing interest from coaches, broadcasters and fans alike. Unlike anecdotal evidence, which are filled with visceral biases, numbers can provide an objective description of player performances or team tendencies. A common feature in collected sport data is repeated measurements on the same observational units. While typical regression models ignore this clustering paradigm, multilevel models explicitly account for cluster heterogeneity and can improve estimates or predictions. We present two multilevel model applications in sport: one comparing face-off skills in women’s hockey, and the other in predicting tennis serve decisions at Roland Garros.
Networking Break 3
11:55am - 12:05pm
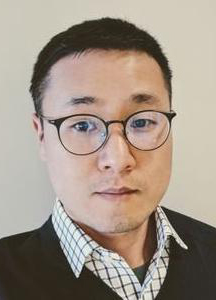
Dr. Yongjin Park (UBC)
What a statistician can do in the wild wild west of single-cell genomics
12:05pm - 1:05pm
How can a statistician survive in the wild, wild west of single-cell genomics? From a naive statistician's perspective, single-cell genomics data may look like other ordinary, existing genomics data matrices. Why are biologists so enthusiastic about this new technology? First, I would like to introduce several statistical learning problems in this newly emerging field in genomics. I will discuss how the frontiers in this battleground meet computational challenges and how they approached and derived satisfactory solutions. In the second half of the talk, I will share personal experience in the world of single-cell data analysis. For a statistical genetics problem, I will demonstrate that single-cell data can be nicely integrated with existing tissue-level, bulk data. For a causal inference task, we will discuss how to leverage a unique data structure of single-cell data on our side. I will conclude the talk by emphasizing the importance of massive data processing armed with useful techniques.